As a part of its open science philosophy, MACRAMÉ is implementing high quality knowledge and data management using state-of-the-art data sharing concepts, approaches and tools and constantly improving research output/data documentation towards full implementation of the FAIR (findable, accessible, interoperable and re-usable) and FAIR for Research Software (FAIR4RS) principles. This is absolutely essential not only for public sharing for reuse but for the internal organisation and management of the complex knowledge flow between the work packages (WPs) to be able to characterise the advanced materials in complex matrices (AdMa@CMs) investigated in the MACRAMÉ Use-Cases. MACRAMÉ’s WP2, responsible for the Development and Advancement of Characterisation- & Test-Methods &-Protocols, and WP4, responsible for the Definition, Demonstration & Validation of MACRAMÉ Methods in Use-Cases,[1] are intensively collaborating to define the needed characterisations, generate flows of information to satisfy the information requirements of downstream experiments, and bring all evidence together for performing Safe-and-Sustainable-by-Design evaluation of the materials along their complete life-cycle. Planning as well as execution of the studies is supported and documented by WP3, responsible for the MACRAMÉ Information & (Meta)Data Harmonisation, Processing & Sharing, which provides the tools for transfer and exchange of all data and knowledge.
Due to the extreme variety of data types collected and generated in the studies, as well as the different starting points with respect to data management at the different MACRAMÉ partners, the data management tools are selected and developed both with flexibility in mind and – more important still – with clear routes to improve the FAIRness of the research output over time at the partner institutions and in MACRAMÉ in general. This FAIRification is not limited to data but extended to all research output including – but not limited to – sampling plans, study designs, in vitro and in silico method specifications, protocols, SOPs, and the data created, as well as guidelines, reports, training materials and publications.
Data Collection & Re-Use in MACRAMÉ
Data from the MACRAMÉ data characterisation techniques will be enriched with information from a multitude of other sources and aggregated for knowledge discovery. This will be done on different levels:
- provide information on materials study design and data analysis stage for specific MACRAMÉ methods;
- test for transferability of analysis approaches from one method to the other; and
- evaluate data aggregation approaches applied in WP4 Use-Cases for automatisation and generalisation.
Collected data will ultimately be applied in existing approaches for grouping and read-across. This will assess the relevance of the data produced in MACRAMÉ for setting the boundaries between nanoforms and grouping. To develop these modified approaches into standard alternative methods, each MACRAMÉ method will define applicability domains and constraints based on and constantly updated with the data produced in the Project including calibration measurements and negative/positive result samples. Full provenance trails to underlying data will be generated over time; to provide a seal of quality for each method, to guide its selection and assay parameters, giving the most reliable result for the material and grouping/read-across application at hand, and ultimately guiding the development of SOPs for standardisation/validation of the method. Grouping and read-across approaches, developed to assist industry with REACH registrations regarding nanomaterials, have been recognised as very useful by ECHA and presented at the OECD, respectively.
Knowledge Generation in MACRAMÉ
Complementing established, validated characterisation methods with the advanced MACRAMÉ methods as input for both regulatory grouping, read-across and risk assessment methods, as well as the for the scoping of Safe and Sustainable by Design (SSbD) initiatives has the potential to increase the predictability and reliability of these methods. While following standardised test guidelines is often enough to trust the quality of the results, the new, advanced methods will have to show the quality by providing information on the robustness, reliability and validity of the method in that they come with highly increased information requirements to be provided with each data point. This data generation process is depicted in Figure 1; it requires a very intense two-way exchange (of information and knowledge) between the WPs and is based on the development of new characterisation methods and their validation of them via the industrial Use-Cases.
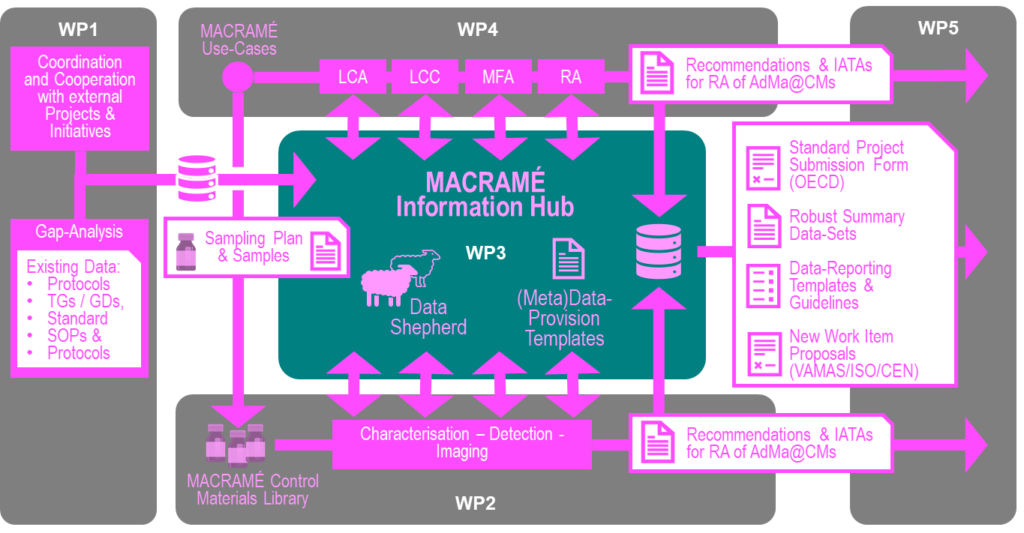
This exchange will occur in the form of materials and sampling plans, study designs translating needs into actions, method specifications describing the measurement principles, protocols and SOPs, and finally the data (raw, transformed and robust summary), all which we will call MACRAMÉ (Meta)Data in the following. For each Use-Case, information is initially generated by WP4 (incl. materials flow analyse (MFA), exposure points, sampling points and protocols, and sample preparation SOPs. Then, the data generation is started with the sampling protocols in WP4, and then continued with characterisation and human toxicity testing (WP2) and with ecotoxicity and life-cycle-based assessments, mass-flow-analyses (MFA) and risk assessment (RA) (WP4); this will be documented with detailed descriptions of the methods, their development and development stages, and the corresponding protocols and SOPs detailing the specific variations needed and the effect of changes to the protocol on the resulting data (e.g. absolute values, uncertainties, repeatability). It is further complemented by gap filling using existing data from public resources identified by WP1, mined, curated and integrated by WP3, and via the modelling approaches of WP3 generating computational data. All of this information will be integrated, harmonised and handed back to the Use-Cases (WP4) for analysis against Life-Cycle Costing (LCC) criteria, and in a condensed (summary) form to WP5, responsible for the Translation into Standards & Policy-Information, and WP6, responsible for Project Coordination, Dissemination, Exploitation & IP Management, as a basis for the development of updated guidelines and standards, and for dissemination of the Project results to target stakeholders. All information will be managed and exchanged via WP3, as depicted in Figure 6, guaranteeing safeguarding of all MACRAMÉ (Meta)Data with full provenance trails, and creating an first implementation of example of the concept of ‘Materials as a Service’-concept (MaaS) for the safety & sustainability of AdMa@CMs. Data to be shared include not only data generated by the fully developed method as used in the Use-Cases of WP4 but every produced data point with their specific protocols from early development, calibration and control measurements, thus collecting knowledge about the applicability domain and reliability of the methods, and forming the basis for the (pre-)evaluation of the methods and for evaluation of their applicability in risk assessment, grouping and read-across approaches.
Data Lifecycle and Data Roles in MACRAMÉ
FAIRification of data, i.e. making data findable, accessible, interoperable and re-usable, is generally accepted as essential for being able to fully exploit the knowledge contained in the immense mass of produced data and is made mandatory in all EU funded projects. However, FAIRification is often still seen as something needed only when the data is shared for reuse, leading to two issues extremely reducing the amount of available FAIR data:
- FAIRification is an external process outside the normal handling of data in the research laboratories, often depicted as the sixth stage of the data management life cycle; it leads to extra work added to the already heavy workload of the researchers.
- First analysis of the data and internal data management cannot profit from the benefits FAIR data brings with it. Benefits such as full access to all relevant information (data and metadata like study designs, method description, protocols, as well as description of quality control, analysis and uncertainty estimation procedures) allow the direct use by other partners and WPs and applying existing standard tools and workflows for further processing (e.g. categorisation and grouping) without the need of time-consuming data transformation to fit the required input format.
In contrast, the MACRAMÉ consortium already agreed at the proposal preparation stage that high-quality data management and FAIRification tasks need to parallel the data production, in order to ensure timely internal data sharing from the study design phase onwards. This was seen as essential to be able to plan the collection of all information needed for characterisation of the AdMa@CMs in the Use-Cases and to realise the complex information flows between the WPs described above. The commitment was formalised by assigning part of the person-months budgets of all data producing and collecting partners to WP3 for covering all data management related tasks. This way, moving the FAIRification task into the earliest stage of the data management life cycle helps to minimise the overhead, even if still extra work is required.
In MACRAMÉ, harmonisation and sharing of (Meta)Data is done as part of the normal experimental workflow. Figure 2 (adapted from Papadiamantis et al.[2]) shows how such an early integration maps onto the data management life cycle, where information is reported in a FAIR way when it is produced, enhanced and completed, while it moves through the life-cycle stages. Clear documentation of the study design in the life-cycle phase 1 “Plan & Design” increases the FAIRness of the data and specifically supports the re-use part of the FAIR concept, since this information gives the data customer (i.e. the first user, as well as a re-user in the form of other partners) important details to understand the (Meta)Data and its reliability. Collection of the data and its analysis in phases 2 and 3 (Collection & Creation and Process & Analyze) are subsequently utilising harmonised and interoperable data reporting formats and workflows directly or are semi-automatically translated into these; this – in turn – guides the provision of the needed metadata. The remaining steps then only need to add more technical information like unique, persistent identifiers (e.g. DOIs), licences and other biographical metadata for improved findability and accessibility.
The MACRAMÉ Registry and Pathway to harmonised Research Output Documentation
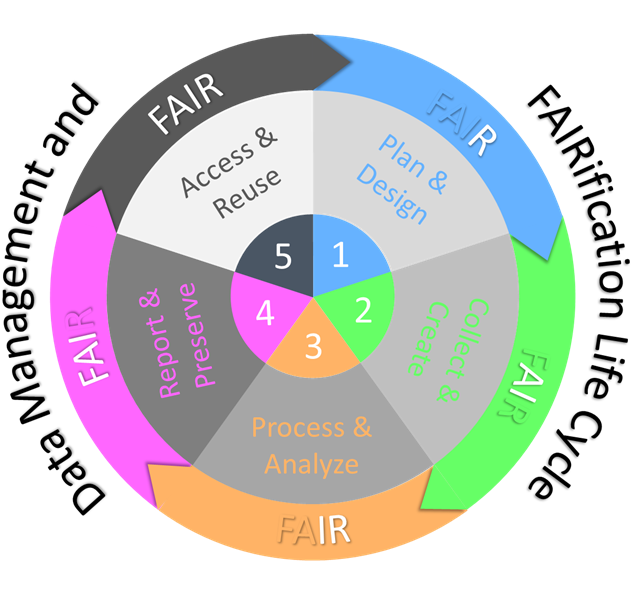
As described above, the MACRAMÉ Control Material Library development and the Use-Cases generate and collect many pieces of information and knowledge as research outputs (e.g. sampling plans, study designs, method specifications, protocols, SOPs, and the data created by them, as well as guidelines, reports, training materials and publications) coming from multiple partners, which need to be shared with the consortium in a timely manner and in parts collaboratively edited. To avoid delays caused by waiting times resulting from the need for agreeing file formats and data models between by all partners, adoption by all partners, or the transfer of data into these formats, the FAIRification actions at this early stage of the Project concentrate on findability and accessibility. This is achieved by the combination of a distributed data storage system with a central MACRAMÉ Registry– the central element of the MACRAMÉ Information Hub – which indexes high-level metadata, like name, type of resource, short description, provider, and documentation completion status, as well as the link to where the resource is hosted. Statuses of resources are ranging from “planned”, over different levels of completeness (i.e. “under development”, “internal review”, and “external review”) to “publicly shared”. In this way, the Registry can already document the need to generate a specific resource identified during the design stage of the Use-Cases or method development and the responsible partner. By changing the status, all partners can then be informed about the progress of creating the resource (e.g. ordering and arrival of starting material, development of a SOP, or collection of data, and location and access routes to the current, most up-to-date version are clearly defined).
Documenting Experimental Design and Execution
Besides data documentation and access via the MACRAMÉ Registry, design of the complex studies and experimental workflows and organisation of the virtual transfer of data but also physical transfer of materials and samples between work packages and partners is supported by visualisation of the flows in the form of instance maps and integrating these maps into the Registry.
Instance maps were first used as organisational structure in the data curation efforts for the NIKC (NanoInformatics Knowledge Commons); they enable users to follow nanomaterial transformations, while capturing necessary metadata. An instance is defined as the nanomaterial in a medium at a specific moment in time. An instance map then represents a flow chart of the nanomaterial fate represented as a directed, often tree-like graph built out of nodes connected by edges represented as arrows to show the directionality. To support the quick generation of such maps, a specific software tool was developed in the NanoCommons Project and now improved and extended in MACRAMÉ. The tool introduced a couple of modifications and new features compared to the original definition of instance maps:
- Besides the five node categories (instance, material, medium, property, and supplementary) of the original approach, transformation protocol nodes explicitly describing the processes leading from one instance to the next, protocols/SOP nodes for experimental details and data nodes were added.
- Information resources can be associated with each node.
These new features especially make instance maps an optimal planning and monitoring tool for the experimental work by creating “mind maps” of the Use-Cases showing material flows, dependencies of experiments, which information is integrated in the SSbD assessment and how.
Nodes can be linked to the resources in the Registry providing structure to the many resources e.g. associated with a Use-Case, giving easy access to all information needed to start the next phase of the study, and in this way, supports the management of the studies and of the overall Project. Within the MACRAMÉ Project, the planning of the experimental work and workflows using the tool has been started as a co-creative training session resulting in partial instance maps with one example shown in Figure 3.
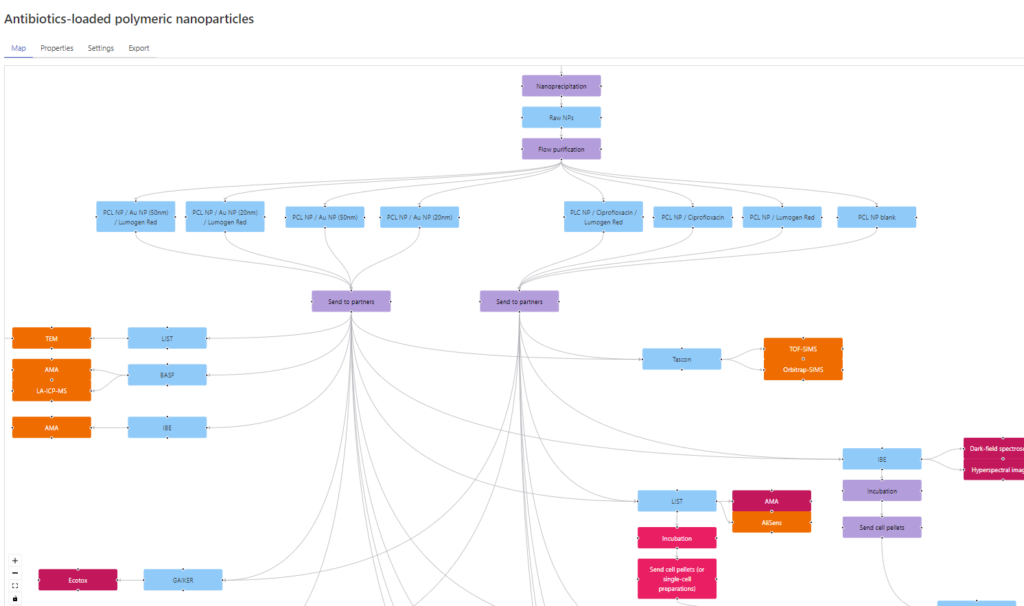
Data Shepherding in MACRAMÉ
To guide the project partners in their Use-Case planning based on instance maps and indexing, uploading and sharing all research output via the MACRAMÉ Registry, a continuous, centralised (Meta)Data Shepherding service was established in MACRAMÉ. For harmonisation and increasing interoperability both within the Project, and with the relevant community activities and the forming European digital materials ecosystem, advanced understanding and knowledge on data management and FAIRification concepts and the available FAIR Enabling and Supporting Resources is required. These requirements resulted in the definition and establishment of data stewards[3] and recently of data shepherds[2]. The latter can be described as an enhanced version of the first, who not only oversees the data management, handling and quality control processes, but can communicate in a clear and simple language with all parties and resolve any misunderstandings[2]. Data shepherds lead the data quality control and FAIRness efforts, selection and/or development of the appropriate data management toolset according to both the data types and the requirements of specific partners, as well as the continuous optimisation of data workflows, including technical developments to facilitate data curation, annotation, and cleansing. In MACRAMÉ, data shepherding was started with multiple trainings and workshops on the use of the MACRAMÉ Registry and the instance map tool (i.e. during the Project kick-off meeting & 1st Project meeting, and through workshops on 15th March and 29th March 2023) and was continued with separate workshops for the five individual Use-Cases, in which instance maps were created. First resources linked to the instance maps were also already included in the MACRAMÉ Registry (mainly using information from material data sheets) and this process will now be continuously, as soon as additional research outputs (protocols, SOPs, data) are generated ensuring timely sharing and establishing the Registry as the route of knowledge transfer between the WPs and then for open and FAIR sharing with all stakeholders. For the latter, further improving harmonisation and interoperability is still needed. As described above, MACRAMÉ concentrated first on findability and accessibility and is sharing the information in the form produced by the individual partners. In a project and especially at the early stages of it, the lack of interoperability can be countered by bespoken solutions for transferring data from one format into another. However, all MACRAMÉ partners are now also working together on defining reporting standards and corresponding SOP/protocol and (Meta)Data reporting formats and corresponding datasets. The data shepherd is supporting this activity by analysing the current data management practises at individual partners and proposing options and tools for the harmonisation of data, improving interoperability and FAIRness. Frequent re-evaluation of the completeness of the provided information for the Use-Cases and for standardisation of the MACRAMÉ methods and output will be performed, and improved reporting guidelines resulting from these activities (e.g. implementing the concept of method-specific reporting) will be proposed to the scientific community and standardisation bodies. In this way, implementation of the FAIR principles at every stage and harmonisation with major European Initiatives (EU NanoSafety Cluster, European Materials Characterisation Council and IndustryCommons) by reusing and extending community interoperability standards (computer-readable and annotated CHADA, CEN CWA 17815 Materials, VAMAS-CODATA UDS) can be guaranteed.
More details about the MACRAMÉ Data Shepherding can be found in its report Information Hub, Data Exchange Format Specifications, and initial Research Output Management Plan (ROMP).
[1] The R&I activities focus on five market-relevant industrial MACRAMÉ Use-Cases, representing three MACRAMÉ Material Families of inhalable carbon-based AdMas of various morphologies and dimensions, beyond spherical particles: (a) graphene-related material (GRM), (b) carbon nanofibres (CNFs), e.g., carbon nanotubes (CNTs), and (c) Poly Lactic-co-Glycolic Acid (nano)particles (PLGA).
[2] Papadiamantis AG, Klaessig FC, Exner TE, Hofer S, Hofstaetter N, Himly M, et al. Metadata Stewardship in Nanosafety Research: Community-Driven Organisation of Metadata Schemas to Support FAIR Nanoscience Data, Nanomaterials; 2020, 10(10):2033, https://doi.org/10.3390/nano10102033.
[3] Mons B. Data Stewardship for Open Science: Implementing FAIR Principles. New York: Chapman and Hall/CRC; 2018, 244 p., https://doi.org/10.1201/9781315380711.